Digital twin and solar flux_
The main objective of this project is to set up control strategies of the heliostat field thus making it possible to generate a flow defined by a user (uniform, gradient, circular etc.). Discover the project through this partnership with the CNRS !
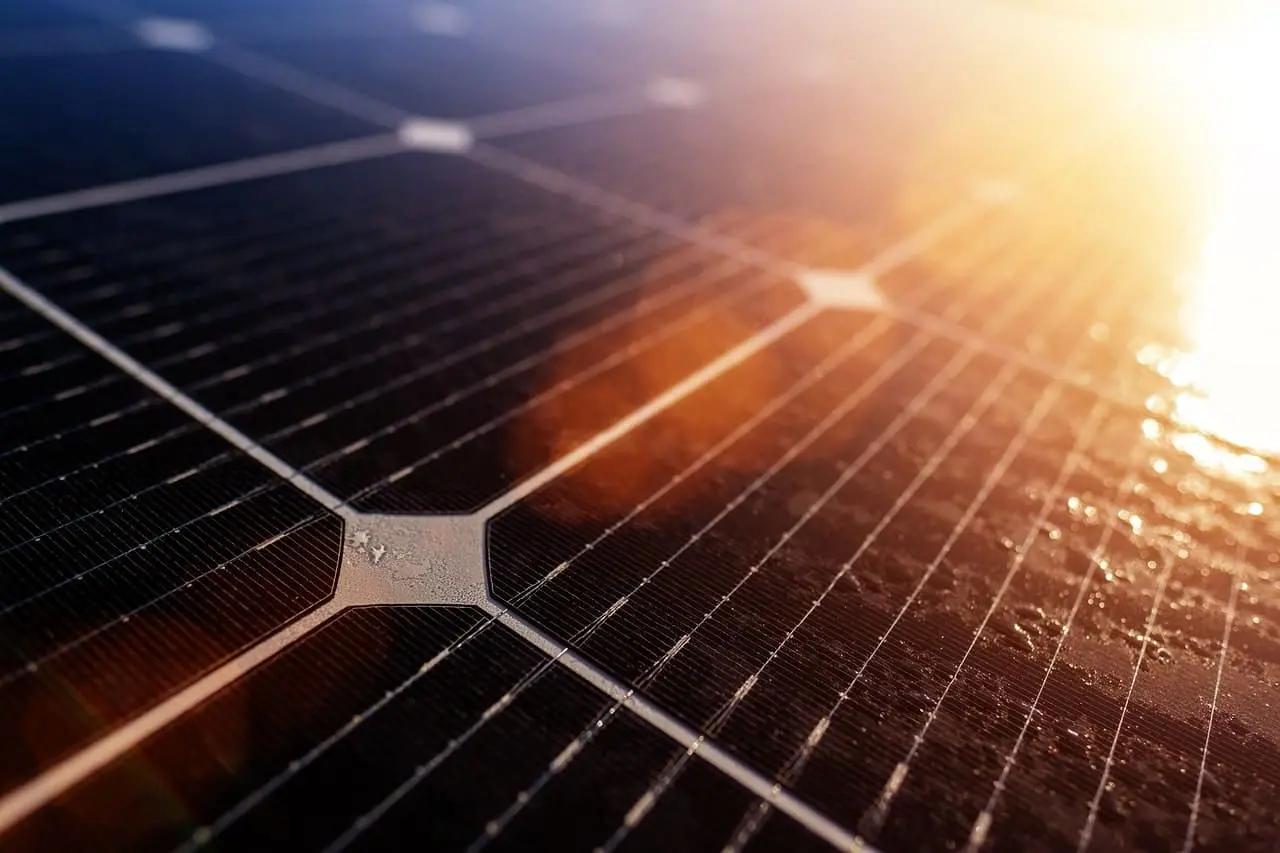
How Machine Learning and the digital twin contribute to the control of solar flux?_
One of the major challenges in the energy sector is the optimization of the implementation as well as the efficiency of concentrated solar thermal power plants. It is in this context that we were able to develop the digital twin of a power plant within the framework of a partnership with the CNRS.
The CNRS Themis team is a laboratory that works on energy production using CSP (Concentrated Solar Power) technology. This technology consists of concentrating the solar flux via a field of heliostats, which can be controlled, at the level of a tower comprising a receiver. This receiver transforms solar energy into electrical energy. As part of the PEGASE project, the Thémis team’s priority was to restore the heliostat field and equip the receiver tower.
Current receiver technologies are robust technologies that can accommodate uneven solar fluxes (presence of hot spots). In order to increase yields, the CNRS wishes to test more efficient receivers, based on ceramics and the latter need a solar flux with no hot spot.
The main challenge of this project is to be able to control the flow in terms of total power absorbed by the receiver but also in terms of spatialization and avoid at all costs hot spots that could cause the receiver to burst.
Digital twin: definition
A digital twin is a virtual model of a complex process or system, updated through a process of capturing and processing data.
At OSE, we have already used this method in a smart shipping tool.
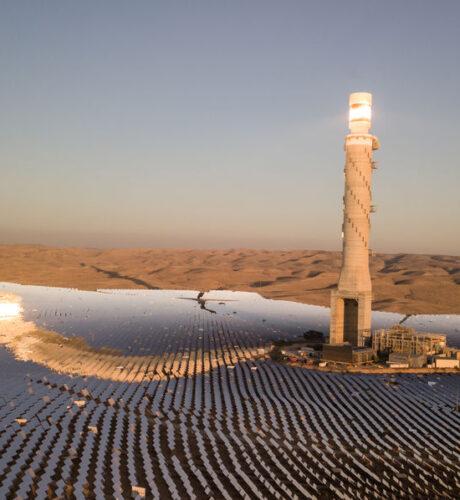
Digital twin and OSE : our missions_
Develop, together with the CNRS team, a methodology for quantifying the solar flux entering the receiver. This method is based on image processing and data fusion technologies with a fluxmeter.
Propose learning models to simulate the flux sent by a heliostat to the receiver, and by the entire heliostat field.
Develop predictive control laws for heliostat field management and flux distribution optimization.
The first phase of this project (solar flux quantification methodology) resulted in the publication of an article in the journal AIP Conference Proceedings.
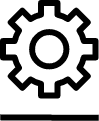
Digital twin in industry_
The benefit of the OSE solution:
Thanks to numerical modeling performed by physical equations and machine learning methods on measured data, we were able to predict the behavior of a system.
We brought digital know-how in image processing, modeling and control strategy
1 OSE developer mobilized
In 6 months
Partnership with the CNRS for 3 years
