How to predict the aging of tunnels?_
How can we predict the aging of tunnels from the following data : geological nature, dimensions of the tunnel, materials of the tunnel as well as the rating assigned during each inspection ? Introducing an example of predictive maintenance !
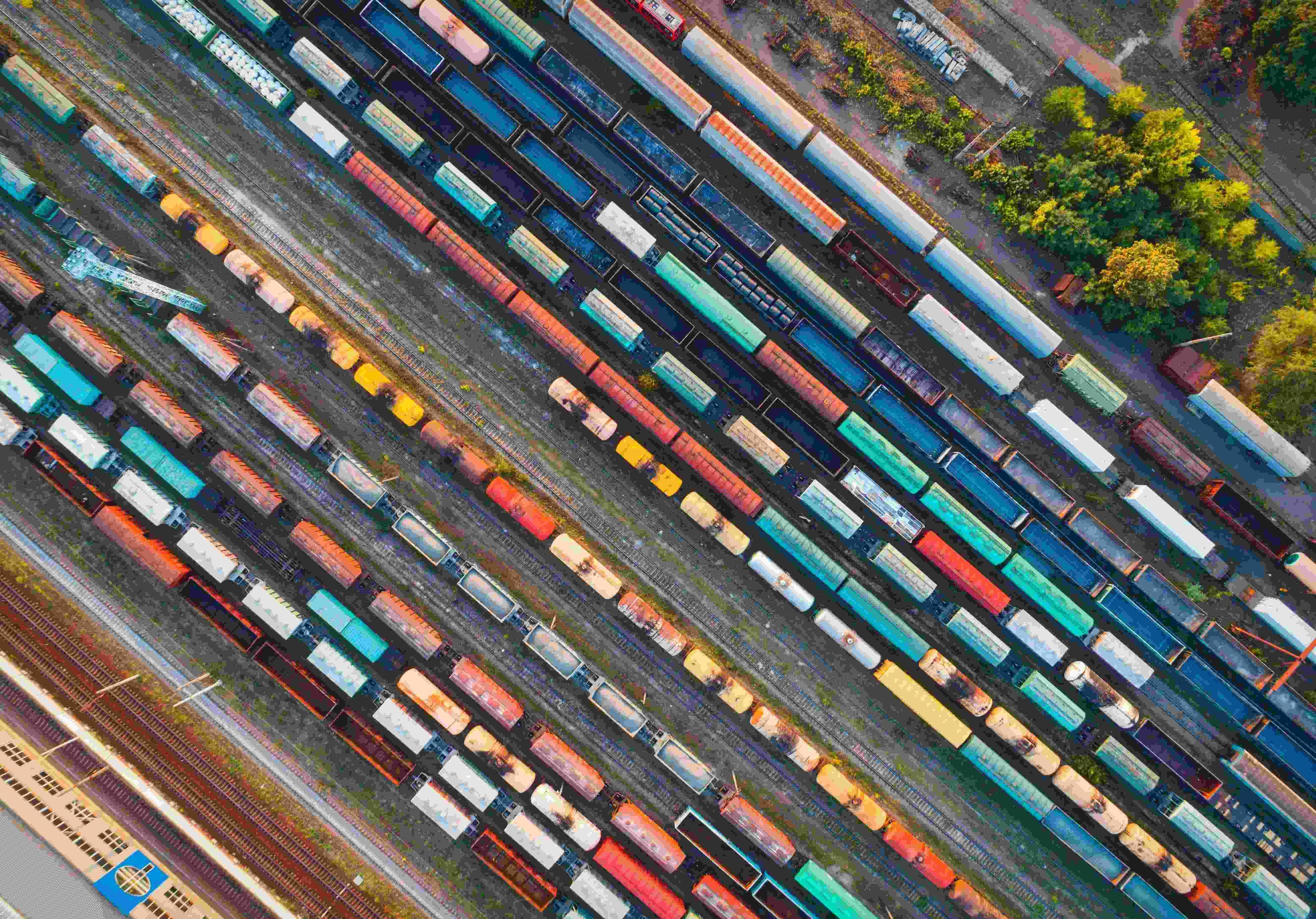
Example of predictive maintenance_
Our client has a portfolio of 1,500 tunnels
The park is aging and maintenance costs are high
Tunnels are inspected and assessed every 5 years on average
By using data from inspection surveys carried out over the past 30 years, the objective is to understand how this fleet of tunnels is changing in order to calculate an aging rate associated with each type of tunnel. This makes it possible to better predict the next moments of intervention, just in time and at a lower cost, to avoid accidents and plan the maintenance budget, thanks to predictive maintenance.
How are inspections carried out?
A tunnel is cut along its length into successive sections of 5 meters each. In each section, the damage is listed (nature, size, location, etc.) and digitized. An overall score describing the state of degradation, called the rating below, is assigned to each section. This dimension varies over time during the various inspections and makes it possible to assess and quantify the deterioration of a tunnel.
The hypothesis put forward by our client is that this coast evolves at a speed depending on various listed criteria: geological nature of the terrain, dimensions and materials of the tunnel, etc.
What is predictive maintenance?
It is a method which consists in anticipating failures, damages, breakdowns by carrying out models of degradation of the systems with the aim of predicting the evolution of a quantity, in this case a coast. Predictive maintenance therefore makes it possible to reduce costs and anticipate breakdowns or damage.
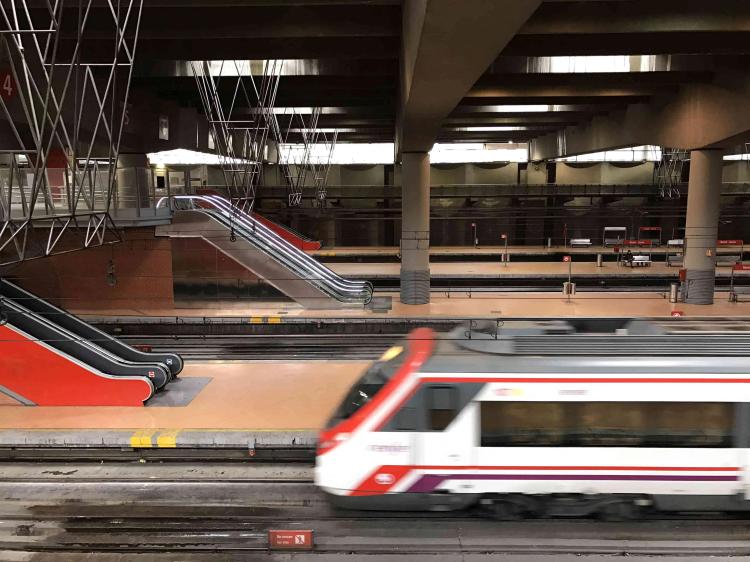
Predictive maintenance : from data to maintenance advice_
Formatting and cleaning of the database: the data used for the analysis come from several sources and were for some collected manually (potential bias or human error)
Analytical study: understanding which factors can influence the aging rate of tunnels and quantifying this influence
Aging rate prediction models : machine learning models based on the most relevant influencing factors
Projection of the coast of the different tunnels in the future to anticipate the works to be carried out
Use of machine learning models to predict the aging rate of a tunnel based on its construction and environmental characteristics.
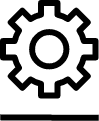
Predictive maintenance and machine learning : Benefits of OSE's solution_
The solution developed by OSE makes it possible to enhance the inspection report database, by projecting the state of degradation of the tunnels into the future.
In this example of predictive maintenance, the applications of such a tool are multiple: fleet maintenance budget planning over the coming years, work prioritization, etc.
2 OSE developers mobilised
In 6 months
Co-development with the client
